- Home
- Resource Center
- Articles & Videos
- The Language Paradox (Or How Mathematics Triumphed Over Language)
28 April 2025
The Language Paradox (Or How Mathematics Triumphed Over Language)

Photo by Google DeepMind on Unsplash
Automation has long been a part of human history. We can cite examples such as the invention of windmills and the wheel in Antiquity, mechanical clocks and the printing press in the Middle Ages, the steam engine in the 18th century, and the emergence of Ford’s assembly lines in the 20th century. Historically, all these inventions followed the basic principle of replacing manual or repetitive tasks with automatic systems that required minimal human intervention. With the onset of the Industrial Revolution in England around 1760, technical and technological advances boosted productivity, and the world changed forever. This marked the transformation from an artisanal model to an industrial-scale production model.
In the mid-20th century, computing and the first computers emerged. Artificial Intelligence was born, along with the field known as “natural language processing,” within which the first language model, Eliza, was developed, and machine translation (MT) began to take shape. In 2015, neural machine translation (NMT) became consolidated and standardized—a more reliable type of MT than its predecessors, based on AI neural networks. In November 2022, ChatGPT 3.5, a large language model (LLM) based on generative AI, was publicly released, sparking the AI hype that continues today. These latest AI advancements are also beginning to impact other services that were not previously subject to extensive automation, such as dubbing, subtitling, and interpreting. This marks a shift from the past, as the tasks being automated are no longer manual but cognitive.
With strong backing from tech giants, the development of NMT and later LLMs has led to an increase in the availability of both free and paid automatic language services, primarily targeted at general users. These services cater to basic, immediate needs—such as instantly translating social media messages—as well as more specific ones. And, as has always happened during AI booms, those developing and promoting these systems make grand promises about their quality and performance, now from a hegemonic position.
Quality Redefined and Exclusion of the Human Expert
Alongside this increasing automation, two significant phenomena are taking place.
1. The term fit-for-purpose is coined, relativizing quality in favor of other factors such as speed or budget when necessary.
2. Automated metrics (one machine evaluating another) are standardly used to assess the quality and performance of these artificial models. This means that human expert translators are generally excluded from such evaluations. The use of these metrics is widespread and appears in assessments and comparisons of various MT and LLM models. Furthermore, since these metrics belong to the computational and statistical fields, translators often cannot fully understand or interpret them, as they lack the necessary computer science background.
Within this framework, the popular imagination—reinforced by the grand promises surrounding AI, persuasive sales rhetoric, and our inherent trust in technology—seems to believe that translation has already been replaced by automatic systems that are as reliable as human work. And this situation presents a major paradox: these language-related automation systems are based on computational and mathematical models from computer science, not linguistics. The role of the human translator as a true expert in the field seems to have been displaced, and mathematics appears to have triumphed over language. In non-professional settings, the general user trusts AI’s promising rhetoric—without the backing of an expert translator.
Can we say that AI advancements have effectively replaced intellectual language tasks, just as manual tasks were replaced by past technologies? The answer likely lies in analyzing how an AI model works and what cognitive processes a human translator performs.
How Language Models Work (And Don’t)
While LLMs produce fluent and sometimes astonishing outputs, they operate on a statistical basis, without actual comprehension. As an influential publication aptly described them, they are “stochastic parrots”—models that replicate language without grasping its meaning. Human communication, on the other hand, involves intention, shared knowledge, and contextual understanding. We infer, interpret, and adjust meaning in real time, drawing from a rich cognitive toolkit that includes memory, emotion, and cultural experience. Machines lack these faculties: they do not understand words, grammar, or meaning, nor do they possess real-world awareness.
The gap between machine output and human communication is often masked using anthropomorphic analogies. Terms like "intelligence," "learning," "neural networks," and even "hallucination" imply that machines process information like humans. But these analogies are misleading. Machines identify and replicate patterns; they do not think, reason, or learn in the way people do. This confusion is amplified by our natural tendency toward anthropomorphism—projecting human traits onto non-human agents. As long as we keep using human-centered metaphors, we risk overestimating what these tools are truly capable of.
What Translation Really Requires
Translation and interpretation involve two essential cognitive processes: understanding and reexpression. Before transferring content into another language, one must first comprehend the message. This requires not only linguistic knowledge but also extralinguistic knowledge—cultural, circumstantial, thematic, and general world knowledge. When a translator or interpreter lacks certain knowledge on a topic, even if they specialize in it, they must do some research. This might involve stepping away from the text to consult other resources that help expand their understanding and ensure an accurate interpretation of the message.
From this perspective, human language is not merely a code that can be deciphered through one-to-one equivalences but rather a communication system deeply embedded in meaning. At times, translation is not about rendering what is said but what is meant, and ambiguities are resolved through context by understanding real-world facts. In the machine translation provided by LLMs, this cognitive process of comprehension is entirely absent. MT results are achieved solely through probabilistic and statistical means, using massive data corpora to recognize recurring patterns.
Translation and interpretation also require other innate human faculties, such as critical thinking and common sense. These skills are essential for problem-solving and decision-making. They enable translators, for example, to detect writing errors or omissions, reconstruct meaning, or grasp communicative intent in cases like subtitles or interpreting speakers who express ideas conversationally and imprecisely.
Are We Really Replacing Language Experts?
AI advancements are undoubtedly leading to increased automation in linguistic services. Can we say that we are heading toward an (almost) complete human replacement, as the most radical tech sectors suggest? Observing how machines work, the answer would appear to be no, since emerging models still fail to address issues such as the lack of communicative intent and deep meaning. Language is synonymous with thought, and, for now, machines neither think nor replicate the cognitive processes that occur in the human mind during translation or interpretation.
Paradoxically, there are indications that some tech giants involved in developing MT and LLM systems may still make use of human translation for certain internal materials or apply automation selectively depending on the context. This underscores the necessity of human intervention when higher quality and precision are required. In professional settings, for all the reasons outlined, humans remain indispensable, and large-scale translation workflows position them as supervisors of machine output (human-in-the-loop). Over two years after the democratization of LLMs with ChatGPT, professional translation still primarily relies on NMT—also AI-based, but with a strong human component, as it includes human translations from translation memories in CAT tools.
The grand promises of achieving general AI are fading as the AI hype subsides. For now, machines lack independent will or human-like intelligence. They are sophisticated tools, but tools, nonetheless. Deciding when, where, and how to use MT should be left to an expert translator, just as no one questions a doctor's expertise in medical decisions. As automation advances, adaptation and IT-related skills become necessary, but so does, now more than ever, the expertise of linguistic professionals where precision is required.
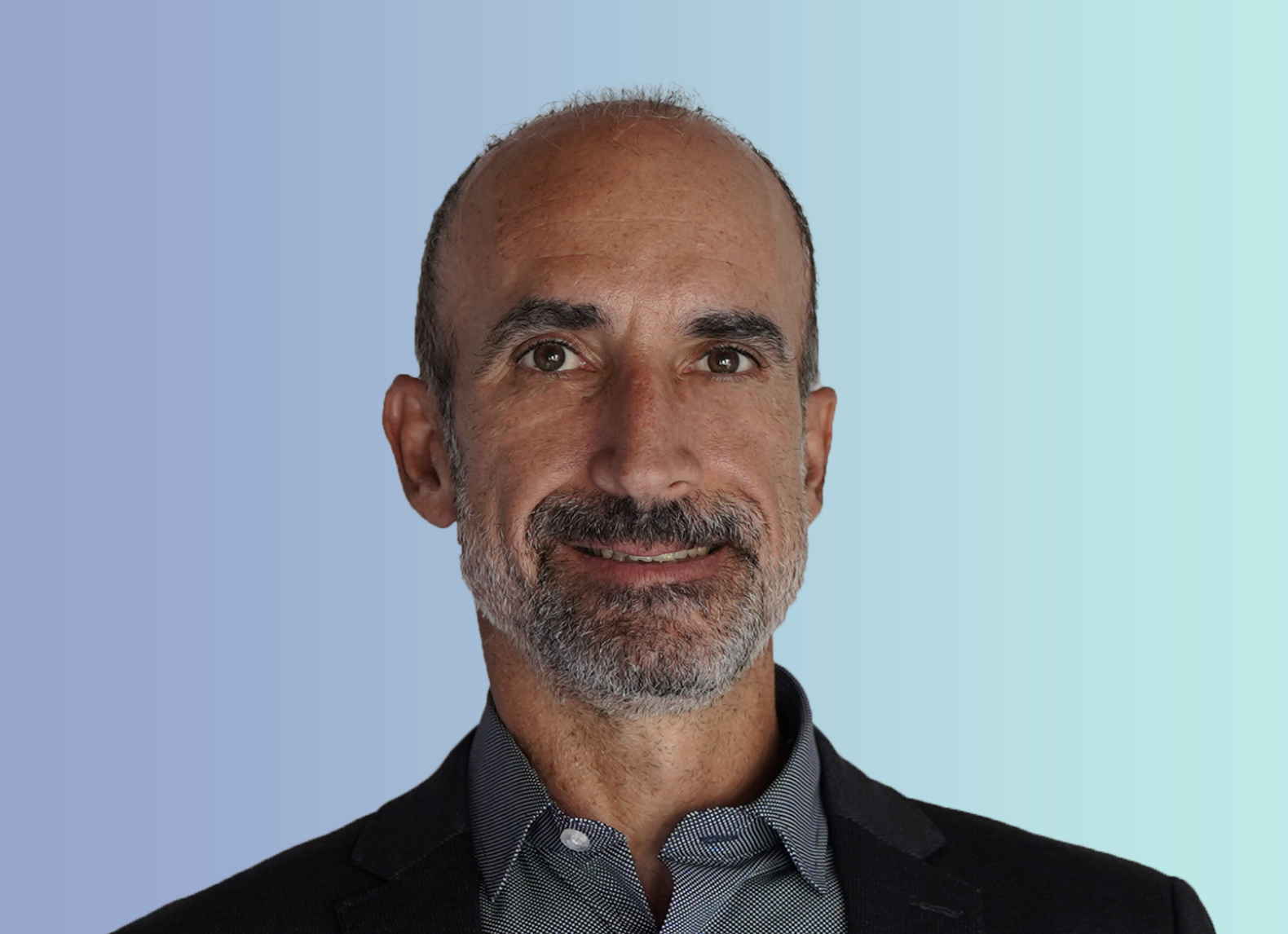
Germán Garis
I am the Managing Director of GeaSpeak, the world’s expert in IT localization for the Latin American region. GeaSpeak is certified under ISO 17100 (Translation Services) and ISO 18587 (Post-editing of machine translation output). We are focused on Spanish.