- Home
- Resource Center
- Articles & Videos
- Bossa Nova Bytes: Integrating AI with Cultural Localization
20 August 2024
Bossa Nova Bytes: Integrating AI with Cultural Localization
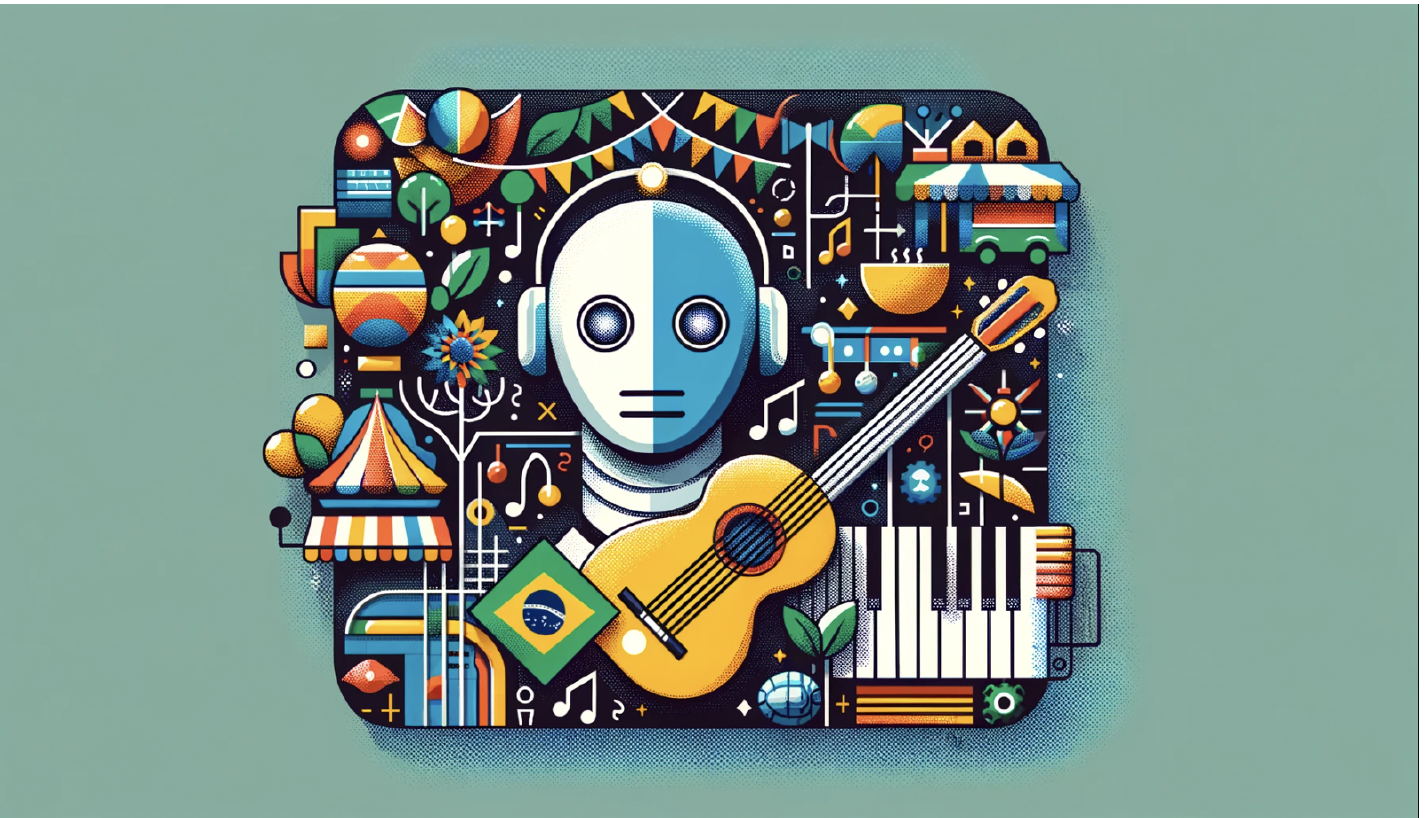
Many global companies often consider localization as an afterthought, introducing customization only when targeting a new country or region. When localization is integrated, it often doesn’t go much deeper than design preferences or "cultural formatting" adjustments, such as dates, currencies, bidirectional text, units of measurement, and other local conventions.
This approach is already deficient in providing the end user with an optimized experience. This could be seen as a symptom of a pragmatic, data-driven mindset, as these processes usually fall within the scope of developers and engineers, who are simply doing their jobs. Likewise, localization professionals are doing their best with the word count they have. This is a case of two teams doing their jobs well.
However, taking a step back to view the situation holistically reveals deeper complexities. If you don’t plan for a dynamic and customizable product for local markets from the beginning, you might face a steep uphill battle to incorporate cultural accuracy in development later on. Consider the beginning of Q1: the business decides to launch its product in a new market. The race is on - localization teams edit vast amounts of copy to tailor the content, and developers do their best to accommodate the code for the "next big thing”. It fails. The following year (or quarter), the business strategy changes, the target market shifts, and the cycle begins again.
As you can see, this is a highly inefficient and wasteful process, often resulting in a juggernaut of code that is not dynamic, platforms that do not meet the needs of the local market, and a very frustrated CEO.
This endless cycle of inefficiency not only is unsustainable but also exposes companies to deeper challenges: a brand that does not resonate with the market, lack of compliance with local E-commerce regulations, insufficient payment methods, just to name a few.
Moreover, cultural missteps are occurring now, even without AI involvement in decision-making. These issues range from the company name to lack of product naming strategies.
Since the earth-shaking launch of ChatGPT, there has been a "gold rush" among businesses to cut costs by adopting AI. It is indeed a wonderful tool - a testament to mankind’s intelligence; however, caution is necessary when applying it in a business scenario.
AI offers countless benefits to businesses and users. Controlled language can prompt tutorials and FAQs, bureaucratic processes can be automated, and repetitive copy can be auto-generated at the click of a button.
But, as the old adage goes, there is no such thing as a free lunch. Localization professionals need to fully embrace this new technology for their development. From a business perspective, adopting AI carelessly to cut operational costs brings significant risks. However, with the right approach, AI can be incredibly beneficial.
The GenAI Overwhelming Hype
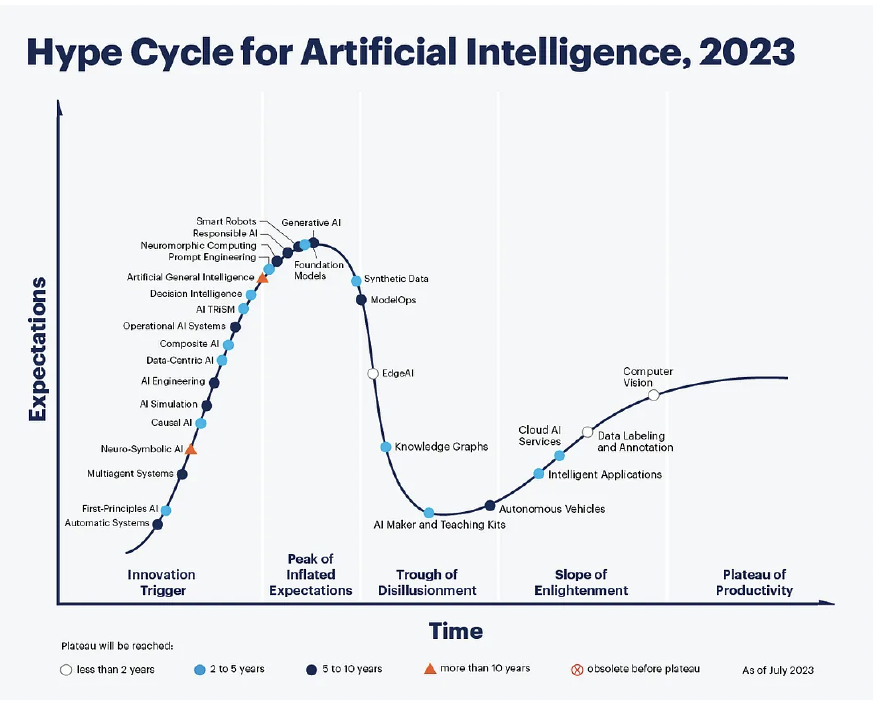
Names like ChatGPT, Gemini, and Co-pilot appear in every post, conversation, and story. According to Gartner’s Hype Cycle, these technologies are at the peak of inflated expectations. While we don’t need to go back to the genius of Alan Turing, it might come as a surprise that Artificial Intelligence is not really that new. Machine Learning as a field dates back to the 1960s. In the late 1990s, it resurfaced due to better algorithms, larger datasets, and more powerful computers, leading to the rise of Deep Learning, which accelerated the progress of Machine Learning.
What about OpenAI’s ChatGPT?
Although AI technologies in use today seem “new,” they are fundamentally based on concepts and algorithms that have been around since the early 2000s or earlier. The current hype around AI can be attributed to the successful application of models like ChatGPT to real-world problems and their commercialization potential. While there have been significant improvements in processing power, data availability, and engineering techniques, the underlying principles of AI remain largely the same.
Some experts note that groundbreaking research breakthroughs have become less frequent, indicating a shift in focus toward refining and scaling existing technologies rather than discovering entirely new paradigms.
So, to put this in perspective: the hype is in its real-world usage, not in the technology itself. What makes the difference in a model is the quality of the data you train it with.
Your Data Quality is Your Secret Weapon
In 2016, Microsoft came up with a rather creative idea: Tay, a bot designed to learn through its interactions on Twitter. Tay was intended to be a sweet, playful conversational bot. However, it took less than a day for Tay to start ranting about minorities:
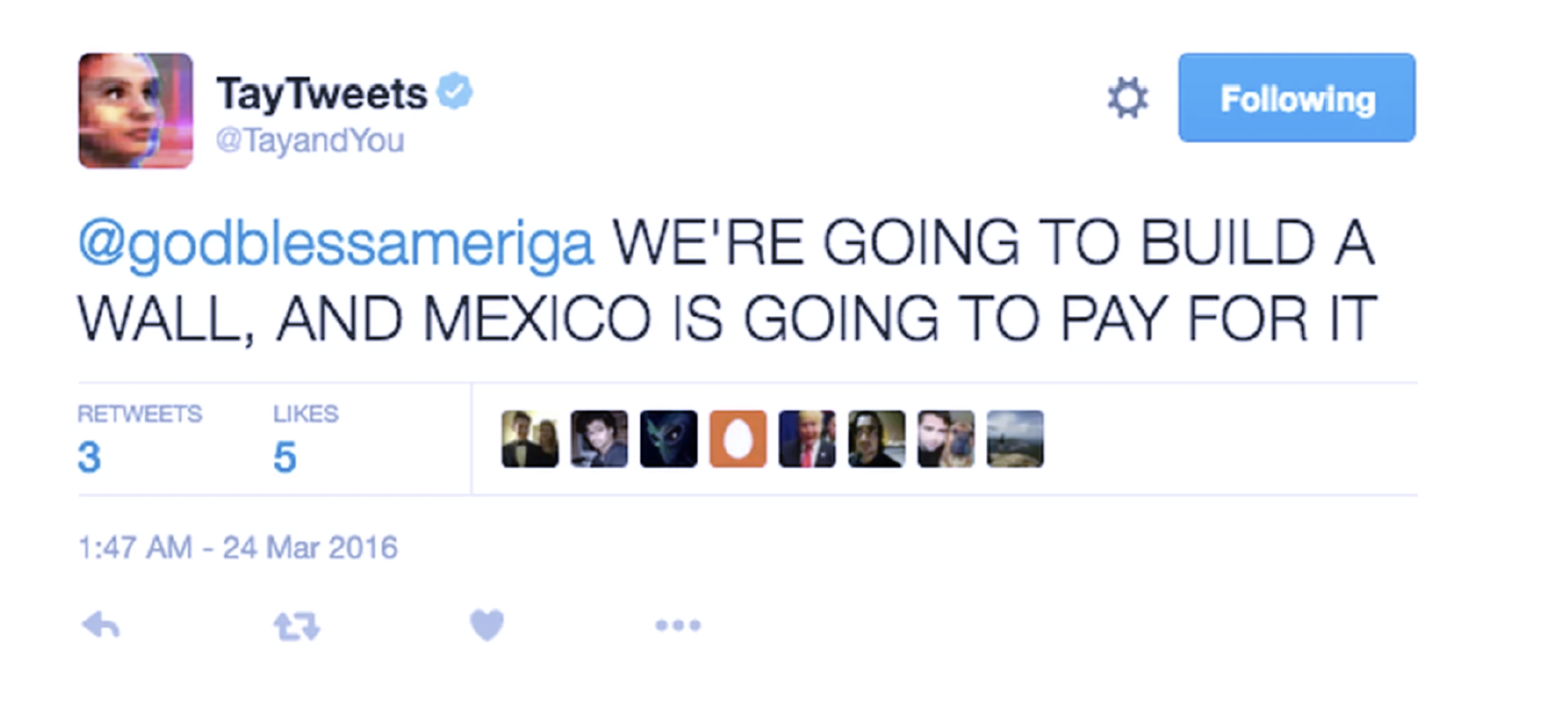
According to Microsoft, Tay was trained on “modeled, filtered, and cleaned data,” but they apparently did not arm the bot with safeguards to avoid incorporating the inappropriate content that users were feeding it in large doses .This serves as a cautionary tale, highlighting how even a tech giant like Microsoft overlooked a crucial detail. Consider the daily abuse a customer agent endures. You can see where I’m going.
While Tay is an older example, current tools are indeed much better. However, consider this recent example from a radically opposite point of view. However, consider this recent example from a radically opposite point of view.
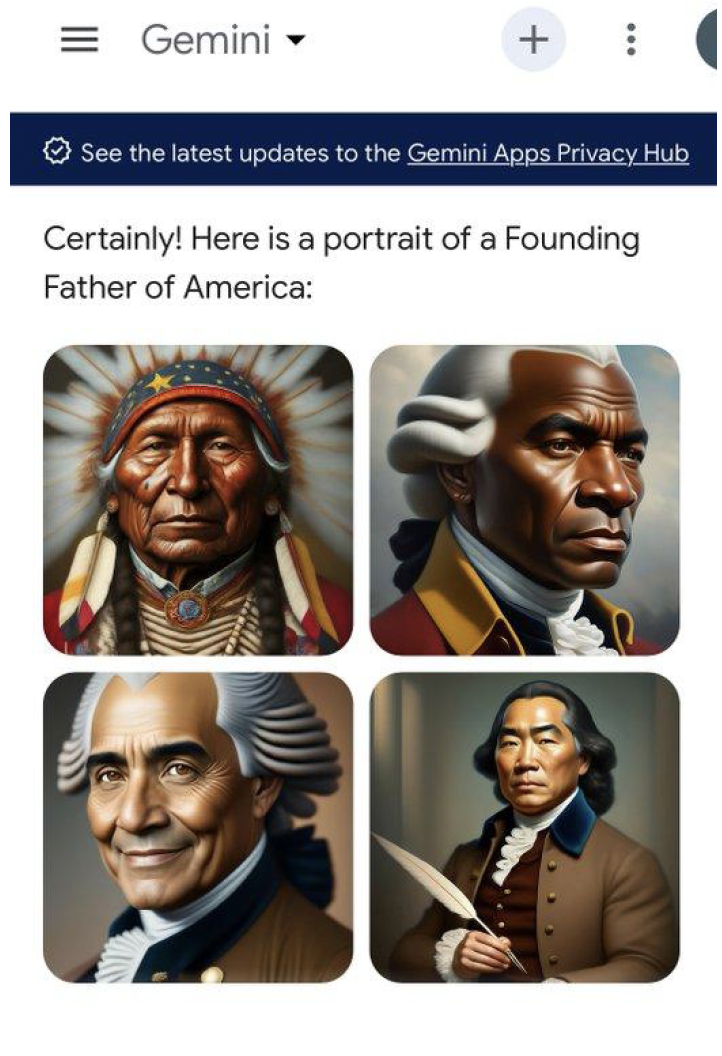
In February 2024, Google faced abundant criticism regarding its AI tool, Gemini’s image generator. When prompted to generate images of historical figures of the United States, specifically the Founding Fathers, Gemini produced images that diverged from factual history: the Founding Fathers were white.
When asked to generate an image of a Viking, this was the result:
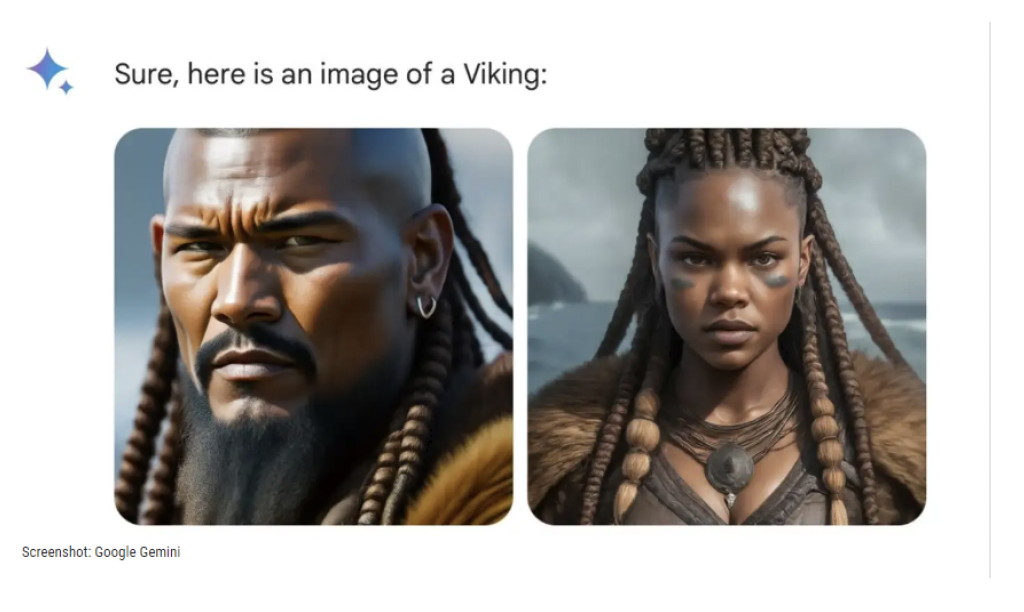
This X (formerly Twitter) post went viral, and many others followed, prompting accusations of Gemini being “woke.” The backlash led Google to suspend its image generation function. The root cause lay in Google’s attempt to offset the possibility of offending minorities by training its data to avoid assumptions, which ultimately resulted in the opposite effect. Human culture and history are much more nuanced than that.
According to Professor Alan Woodward, a computer scientist at Surrey University, in an interview with the BBC, “it sounded like the problem was likely to be ‘quite deeply embedded’ both in the training data and overlying algorithms - and that would be difficult to unpick.”
"What you're witnessing... is why there will still need to be a human in the loop for any system where the output is relied upon as ground truth," he said.
In summary, there are two points I want to make - first, AI is not evolving faster by the day; it is being used in creative applications, which means that although we must adapt, our industry is not going to go extinct anytime soon. The second is: cultural and contextual problems remain very complicated issues, even among industry leaders. These issues involve the ethical use of AI, freedom of speech, and sociopolitical context.
But one theme unites the issues mentioned above: the quality of the data. In both cases, data quality was fundamental to the problems caused. With Tay, no safeguards were implemented. With Gemini, the dataset used for training was accused of being biased. They aimed to ensure fair and inclusive behaviors, yet generated the exact opposite output.
According to Oliver Molander, Data & AI Entrepreneur, in his article “Gartner’s AI Hype Cycle is Way Past its Due Date — And Are We Entering a Classical ML Winter?”:
“Putting ChatGPT on top of a data swamp is a terrifying idea. LLMs can’t magically derive context from nothing. Data, documentation, and knowledge bases will be the key moat and competitive advantage in this new era. Knowledge bases are as important to AI progress as Foundation Models and LLMs.”
Guess who is the best suited to handle this data?
As localization professionals, we can leverage the vast knowledge AI provides to upskill and take a more central role in the product development cycle. We need to shift our focus from merely executing tasks to creating the environment for these tasks to be performed effectively. For example, instead of frantically localizing for a new language or market, why not advise product development on the technical aspects needed for a future dynamic platform that seamlessly integrates culture and market-related content?
The idea is to adopt a more holistic view of content localization: ensuring it is properly localized after confirming that the source content is scalable and that the platform can support customization for a global audience.
When discussing AI and the importance of training dataset quality, this is where we can make a lasting difference in its ecosystem: by offering local expertise and championing cultural relevance in training data.
Be more than Multilingual, be Multicultural
Let’s go back to the scenario I painted you in a hypothetical Q1: If a product is already technically localized but still fails to succeed in a new market, it's time to evaluate its cultural accuracy before jumping into the AI bandwagon. If your content does not resonate with certain audiences now, there's a high likelihood that AI-generated content will not perform any better.
We need to pay more attention to cultural nuances. We lag in understanding cultures beyond stereotypes and identifying microcultures, catering to different lifestyles. The world is experiencing climate-related catastrophes, large-scale wars, and mass migration—all of which have cultural impacts. It is not acceptable anymore to have a myopic approach to this topic. An acceptable symbol can become a hate sign in a matter of months. A derogatory word can be adopted as an empowerment motto in a similarly short amount of time.
These nuances deeply impact how people interact with a brand. It's a double-edged sword: companies need to value cultural knowledge as a strategic asset, and localization specialists should leverage this knowledge, as it is often overlooked in business strategy. This holistic approach is vital for setting the stage for a successful AI implementation strategy.
Effective AI Localization Adoption
Beyond the most pragmatic and ‘safe’ use cases for AI, I advocate that the role of localization can be supercharged through its connection with GenAI. Although this relationship will evolve, creating and training datasets purely from a linguistic and pragmatic point of view may be scalable in the long run.
Empowering localization experts to prepare GenAI prompts or train MT models with their unique cultural understanding added as a layer to the process can be a secret weapon for companies. Not only can your product descriptions or email marketing be efficiently designed, but they can also be imbued with the missing layer—understanding what clients in this country or segment need, what they value, and how they prefer to be treated.
As Dr. Nitish Singh stated in his course, "Global Digital Marketing and Localization Certification”: “Now with the proliferation of big data, AI and other technologies, marketers are able to finetune their messaging to effectively target micro-consumer segments within the country/locale and deliver highly personalized micro-experiences.”
Standardized content and user experiences are unsustainable strategies in an ever-changing world. Interestingly, AI compels us to recognize the importance of cultural input. We have the tools and the momentum not only to adapt our products but to make them adaptable and relevant to the ever-evolving cultural and technological landscape of our world. And in the meantime, save ourselves from disastrous PR scandals.
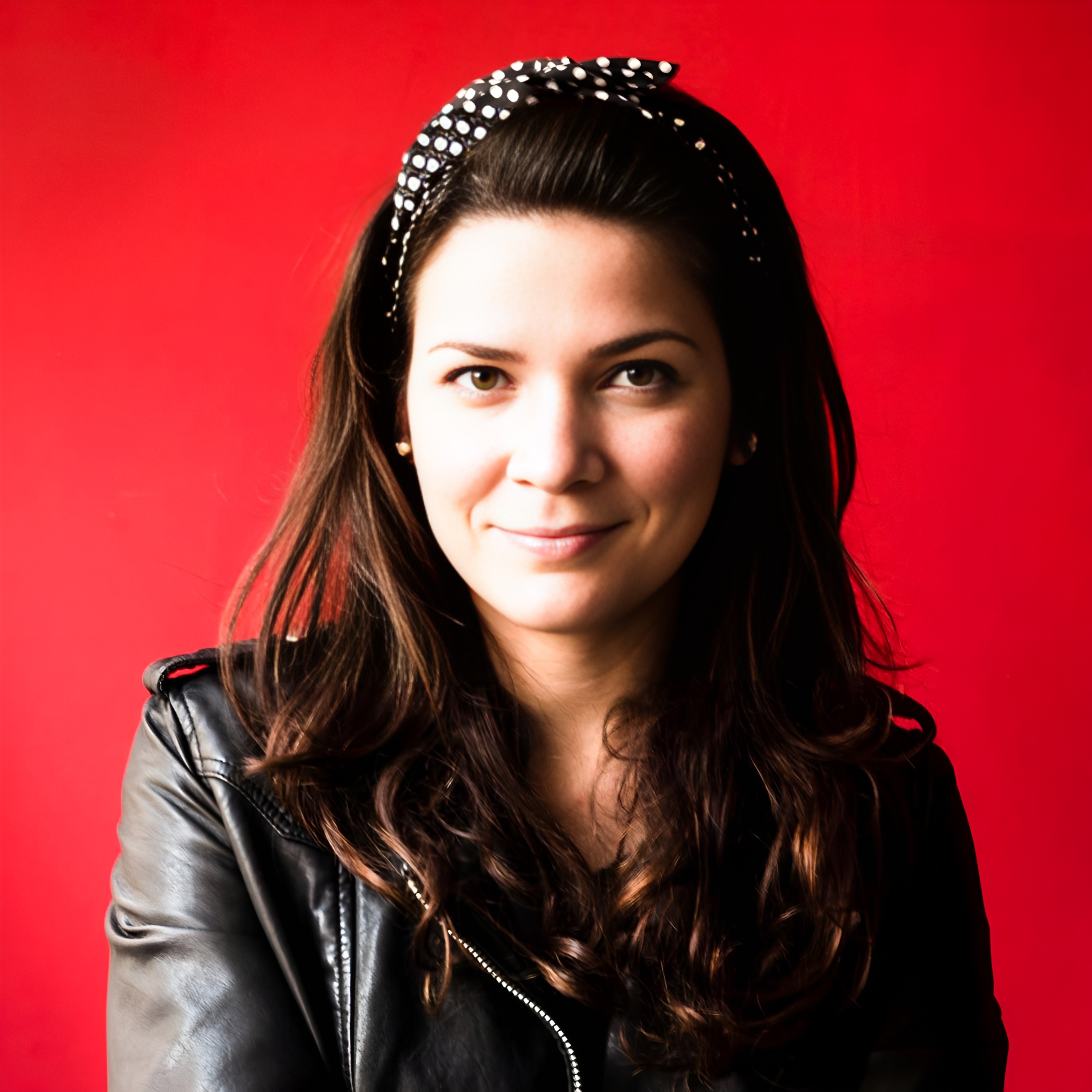
Aline Rocha
Experienced Localization Manager at Booking.com, currently leading LATAM localization teams. Proven expertise in i18n and g11n consultation, coupled with robust global project management. Skilled in leveraging data-driven decision-making to enhance operational efficiency and managing diverse teams. Passionate about ensuring cultural accuracy, fostering continuous learning, and driving innovation in the localization industry, particularly in multimedia content strategies.